Abstract
Wearable sensors, combined with machine learning, the Internet of Things (IoT) and big data have the potential to revolutionise cardiovascular health monitoring. These sensors provide real-time data on heart health, empowering individuals and healthcare providers by moving towards the early detection of cardiovascular conditions. With an ageing population, remote monitoring of these conditions offers independence and reduces healthcare costs. While challenges like data security exist, integrating wearable sensors into clinical settings promises enhanced patient care and treatment outcomes. This combined technology, utilising wearables, artificial intelligence, machine learning, big data and the IoT heralds a new era in cardiovascular disease management, with an emphasis on prevention, early detection, and personalised care for better patient outcomes.
Introduction
As the leading cause of mortality both globally and in New Zealand, cardiovascular diseases (CVDs) have evolved into an ever-present issue deeply ingrained within society today. CVD encompasses a spectrum of medical conditions related to the heart and surrounding blood vessels. Among these, ischemic heart disease, stroke and heart failure stand out as the most profound contributors to the high mortality rate associated with CVD.1
Typically, a cardiovascular event such as a heart attack occurs due to inadequate blood delivery from the heart to various body regions, impairing their normal functionalities.2 Lifestyle factors, specifically a healthy diet and exercise regimen, alongside genetic predispositions can all aid in decreasing one’s risk of developing CVD.3 Furthermore, the early detection of these conditions can aid in decreasing one’s risk of morbidity and early mortality.2 CVD not only detrimentally affects the livelihoods of individuals, but also poses a significant financial strain on the healthcare system, particularly given the challenges posed by our ageing population. In fact, the World Heart Federation has estimated that the global cost of CVD treatment will increase from approximately USD 863 billion in 2010 to USD 1,044 billion by 2030.4
Long term studies have demonstrated the existence of a relationship between a high resting heart rate and adverse clinical outcomes related to CVDs.5 Nevertheless, as of late, the diagnosis of CVDs has proven to be a costly and time-consuming endeavour, involving multiple tests and evaluations within a hospital setting. Commonly used diagnostic tests involve electrocardiograms (ECGs/EKGs), which measure the heart’s electrical activity, MRI and CT angiography, which provide detailed views of the heart and surrounding vasculature as well as blood tests to assess cholesterol and interleukin/cytokine levels.6 Whilst these technologies are frequently used in hospital settings, they often fail to address one of the root causes behind the high prevalence of cardiovascular diseases: the lack of emphasis on early disease detection in asymptomatic individuals and self-management of symptoms to prevent irreversible damage to the cardiovascular system.
Given these limitations, a pressing need therefore emerges for alternative diagnostic technologies that offer greater accuracy, precision and less variation between individuals. Wearable sensors, a transformative, compact and user-friendly technology, provide real-time data on a variety of biological and physiological parameters, enabling proactive management of one’s cardiovascular health.7 The seamless integration of wearable sensors with artificial intelligence (AI) and machine learning (ML) holds promise for more precise diagnostic and prognostic models, thus enhancing the power of early detection in the treatment of CVDs.8
The ageing population
Due to medical advancements over the last 100 years, individuals are living longer than ever. By 2050, it is projected that the global population of individuals aged 60 years and older will double, reaching an estimated 2.1 billion.9 These medical breakthroughs, encompassing enhanced treatments for chronic diseases, new surgical techniques as well significant advancements in the pharmaceutical industry, have all contributed to individuals living longer and healthier lives.
The financial burden placed on the healthcare industry by an ageing population is formidable, yet it presents an opportunity for incorporating long-term monitoring of physiological parameters conveniently within one’s home environment. Wearable sensors and other digital technologies hold great promise for transitioning from traditional clinical based medical practices to remote healthcare, revolutionising geriatric care through enabling independent monitoring of cardiovascular conditions.10
Moreover, remote monitoring of cardiovascular care may help play a crucial role in the early detection and prevention of serious health issues, enabling clinicians and healthcare providers to intervene before serious complications arise. As an example, wearable devices can detect irregularities in heart rate or fluctuations in blood pressure, alerting both the individual and their healthcare team so that potential problems can be addressed swiftly and without the need for a clinical setting.11, 12 In the long run, this approach to cardiovascular monitoring can lead to better patient outcomes and reduced healthcare costs for individuals with chronic illnesses.
Additionally, wearable sensors have the potential to enhance the quality of life for elderly individuals by promoting a sense of independence and autonomy that the traditional rest home living approach to geriatric care does not offer. By empowering individuals to monitor their health conditions from the comfort of their homes, wearable sensors may aid senior citizens to maintain a sense of control and dignity as they age. This shift towards remote monitoring may additionally reduce the burden on caregivers and healthcare providers, allowing them to focus their time and resources on individuals with the greatest need for around the clock monitoring and care. With a global shortage of healthcare providers, this becomes especially important in the years to come. The 2016 Global Strategy on Human Resources for Health: Workforce 2030 projected that there will be an estimated shortfall of 18 million healthcare workers worldwide by 2030, particularly in low-middle income countries.13 In a 2023 report by Te Whatu Ora, it was revealed that there is an estimated shortfall of 1,700 doctors in New Zealand, with that gap projected to increase to 3,400 by 2032.14 A shortage of healthcare workers, an issue which is prominent globally, is further aggravated by physicians increasingly migrating to higher income countries in search of better working conditions and higher salaries.15 The adoption of remote healthcare technologies offers a crucial solution to bridge the gap in healthcare accessibility and delivery, ensuring that individuals, regardless of their location, can access vital medical services, even in the face of a healthcare workforce shortage.
Current state of the art technology and commercially available products
Over the past decade, society has seen a drastic increase in the number of consumer technologies with the capability to monitor cardiovascular parameters such as heart rate, blood pressure and percentage oxygen saturation. Clinicians are often presented with data recorded on such devices alongside queries and concerns by an individual in regards to their physical health. Nonetheless, the accuracy of these devices and their viability as clinical diagnostic tools remain subjects of ongoing debate. Yet, one cannot overlook the user-friendliness and convenience that these devices provide, in comparison to hospital-based technologies used in the clinical diagnosis of CVDs.16
First generation wearable sensors, mainly watches, primarily focused on monitoring of biophysical parameters such as physical activity, heart rate or body temperature. In recent years, there has been a notable shift towards non-invasive or minimally invasive technologies featuring biochemical and multimodal monitoring, marking the shift towards truly personalised healthcare for the individual. These second-generation wearable sensors are most commonly defined by their utilisation of biofluids, employing the use of biorecognition elements to detect the presence of specific analytes into a signal which can be used by the sensor for analysis. Similar to first-generation devices, second generation wearable sensors come in various non-invasive formats including tattoos, contact-lenses and tooth-mounted films as well more invasive forms such as microneedles and injectables.17
One of the most widespread technologies used in cardiovascular care is long-term cardiac troponin monitoring.18 The release of cardiomyocyte components, for example, the biomarker cardiac troponin (cTn) occurs when muscle cells of the heart endure damage. Due to its high sensitivity, cTn serves as the biomarker of choice for clinical diagnosis of myocardial infarction (heart attack). In comparison to historically used clinical biomarkers for the diagnosis of heart attack such as creatine kinase (CK) and its MB-isoenzyme19, lactate dehydrogenase isoenzymes, inflammatory markers like C-reactive protein (CRP)20, and myoglobin, cTn is highly specific for myocardial injury, as its release denotes serious and probably irreversible injury.21
Whilst molecular biomarkers enable highly sensitive data measurements and provide valuable insights into the cardiac health of an individual, the user-accessibility and ability for widespread use is not as profound as we see for physicochemical sensors. A recent state of the art physicochemical sensor is that of the AT-patch, developed by the South Korean company, ATsens. The innovative design of this wearable ECG patch enables real-time transmission of vital signs such as heart and respiratory rate as well as body temperature. Whilst these patches are compact in their design, their accessibility is still an ongoing issue as the cost of an individual patch with a 14-day use period is almost NZD $242. User accessibility of these patches, especially in low-income households, thus may not be accessible at present.3 An example wearable ECG sensor such as that shown in Fig. 1 is composed of a flexible, highly adhesive polyethylene terephthalate (PET) film, onto which the temperature sensor and ECG sensors are printed. An equivalent circuit is established between the highly adhesive and conductive polymer and the skin to ensure accurate signal transmission. The integration of these components enables precise monitoring of temperature changes and ECG signals in a non-invasive, comfortable and cost effective manner.22
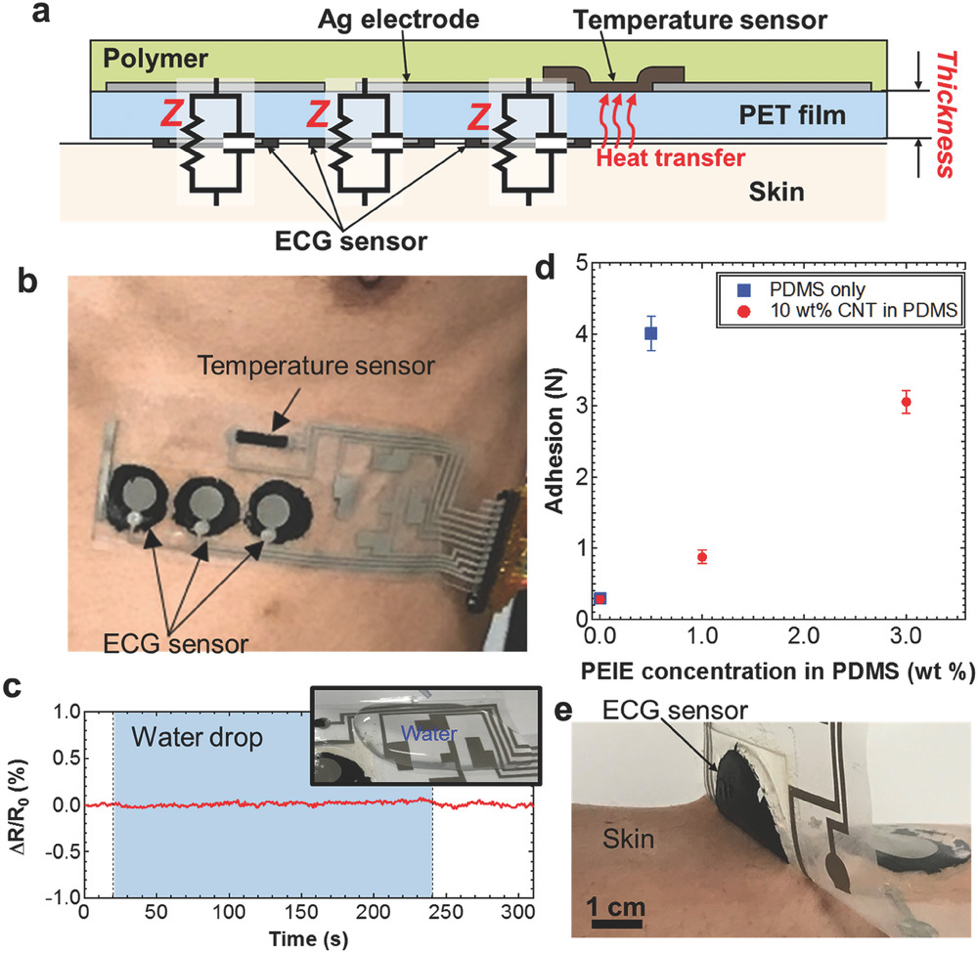
The Oura Ring (Fig. 2), a sleek wearable device that tracks various biometric parameters, offers a promising solution to these ongoing accessibility issues in healthcare monitoring through a simple upfront investment of NZD $500, followed by a monthly subscription of NZD $10.23 This eliminates the barrier of ongoing financial constraints, making monitoring of cardiovascular health more accessible to individuals across various socioeconomic backgrounds.
With its advanced sensor technology, the Oura Ring possesses the ability to sense more than twenty biometric parameters, including, but not limited to, blood oxygen (SpO2), respiratory rate and heart rate. The Oura Ring operates through utilising three key sensors: an infrared photoplethysmography (PPG) sensor, a negative temperature coefficient (NTC) sensor and a 3D (tri-axial) accelerometer. PPG sensors emit light through LEDs, to capture reflections from arterial pluses with a photodiode, enabling precise measurement of heart rate and respiration. NTC sensors provide a decrease in resistance with an increase in temperature, such that when combined with an electronic circuit provides a means of continuously monitoring night-time skin temperature over a very wide range.24 This can therefore be used to provide insights into the body’s recovery from illness, hormonal fluctuations or potential sickness. The 3D accelerometer records daily activity such as step count as well as sleep activity such as restlessness and sleep stages.25 Through the combination of these three sensors, the Oura Ring provides users with detailed insights into their health. Whilst not currently used in clinical practice, clinical trials are currently being conducted to validate these technologies against gold-standard clinical protocols.

The use of the internet of things (IoT) in wearable sensor technology
The internet of things is responsible for connecting smart devices such as watches, phones and other technologies. Much of the wearable sensors used by individuals today are connected to the IoT, enabling real-time insights into the well-being and health of an individual. In general, the signals detected by wearable sensors are transmitted to mobile devices such as smartphones through Bluetooth or Wi-Fi connections. Subsequently, the smartphone then serves as an intermediary device, pre-processing the sensor data before transmitting it to a medical cloud-based platform via a mobile network. Upon arrival at the medical cloud platform, the data then undergo analysis before being stored for further use. Insights derived from the analysis of this data are then relayed back to the smartphone, enabling users and their healthcare providers to access information regarding their physiological parameters and associated health conditions (Fig. 3).26
Devices connected to the IoT capture vast amounts of data from the sensors embedded in wearable devices. Moreover, due to the large variety of IoT connected devices, there is substantial heterogeneity in the data obtained and thus the formats, features and sources of the data can vary. Data heterogeneity has made the quality, processing and storage of data a challenging task.27 As a solution to these issues, machine learning algorithms are now being employed to process and analyse these vast libraries of data, in the process identifying patterns, anomalies and correlations.28 Through sophisticated data manipulation techniques, machine learning models are able to extract viable information which can then be used to provide insights into the health of a patient. Through the constant evolution and refinement of machine learning algorithms, these systems are constantly improving in regards to their accuracy and effectiveness, which as a consequence leads to enhanced patient-specific predictive insights.29
The integration of the IoT into wearable sensor technology thus offers numerous benefits in the realm of remote healthcare monitoring. Not only does this combined technology provide a centralised and scalable infrastructure for storing and analysing vast amounts of patient health data, but it also ensures that individuals’ health information is securely managed and easily accessible.30 This integrated technology not only improves the efficiency, cost and effectiveness of healthcare monitoring but also promotes greater autonomy and engagement among individuals in managing their health. As this technology continues to evolve and improve, the potential for IoT-connected wearable sensors to revolutionise healthcare delivery and improve patient outcomes, particularly in ageing populations, is vast.
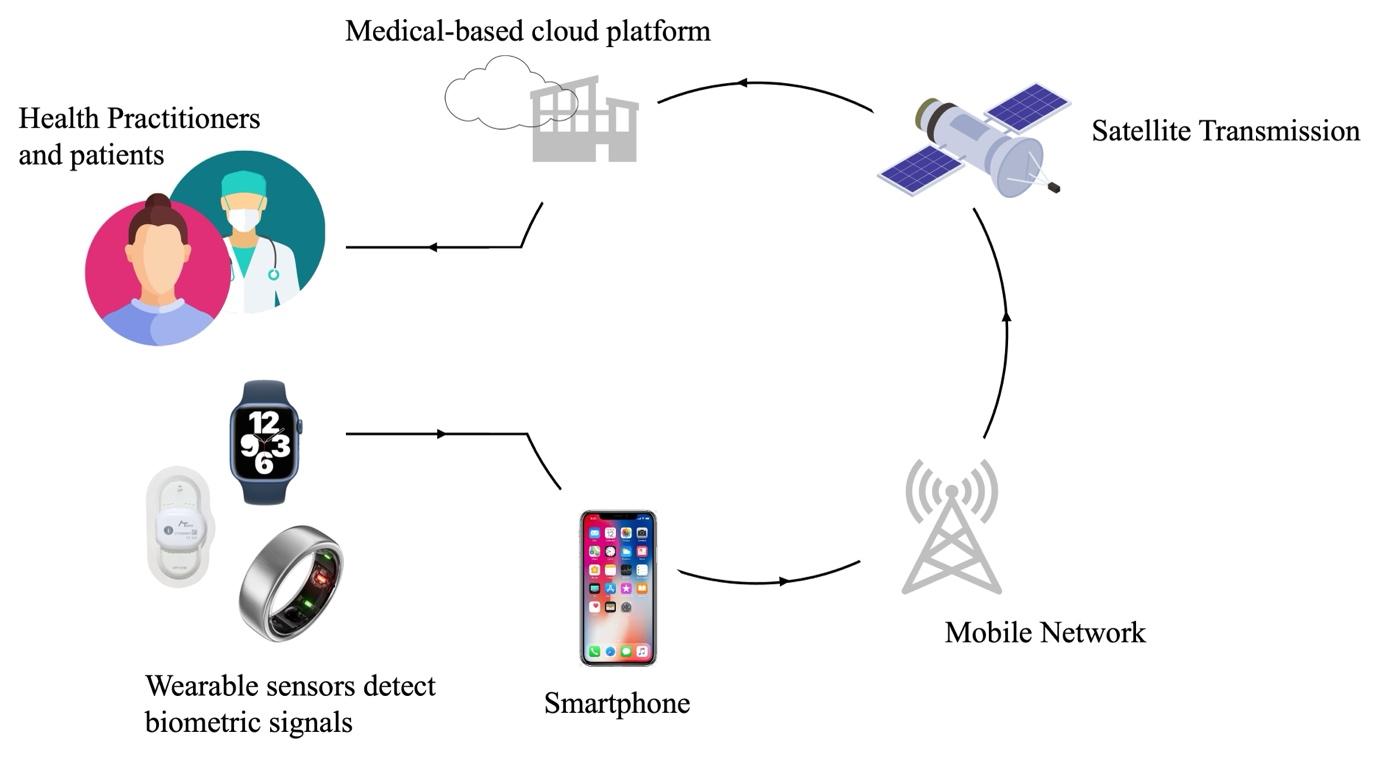
Big data in the diagnosis of cardiovascular disease
‘Big data’ refers to large data sets containing various types of patient information such as medical history, demographics, lifestyle factors and clinical test results are available for evaluation by machine learning algorithms.31 The integration of big data technology and machine learning algorithms into CVD treatment holds promise for enhancing the accuracy of early detection for a range of cardiovascular conditions.
Current wearable devices such as Fitbit, Garmin and Apple watches come equipped with built-in photoplethysmogram (PPG) based sensors, capable of detecting fluctuations in blood volume in the microvascular tissue bed using light-emitting diodes and photodetectors (Fig. 4).11, 32-34 Despite showing a high level of accuracy, a low mean average percentage error (MAPE) and strong correlation coefficients compared to a reference device (ECG leads), all PPG-based devices tend to underestimate heart rate.35 This presents an opportunity for the integration of big data and machine learning algorithms to enhance the diagnostic capabilities of these devices and improve the early detection of cardiovascular conditions such as arrythmias.

However, because wearable devices monitor physiological parameters under non-standard conditions, many of the signals collected are corrupted due to factors such as body movement, artifacts, noise and the degree of contact of the device with the skin.32 To combat these issues, various algorithms have been proposed, utilising signal processing or machine learning for the purpose of signal quality assessment to improve the accuracy and reliability of these devices.36, 37
Machine learning techniques leverage complex algorithms to analyse vast amounts of patient data or ‘big data’, including medical imaging results, health records and genetic information, to identify patterns indicative of various diseases or conditions.
In contrast, molecular computational diagnostics involve the analysis of biological molecules such as DNA or proteins, using computational methods to detect diseases at the molecular level.38 By examining genetic variations or biomarkers associated with specific conditions, molecular computational diagnostics offer precise and personalised insights into the cardiovascular health of an individual.39
Together, big data, machine learning algorithms and molecular computational diagnostics can enable tailored treatment strategies, earlier detection of cardiovascular events and more accurate diagnoses, ultimately leading to improved patient outcomes.
Integration with artificial intelligence and machine learning
The human body can be classified according to various interconnected systems, such as the circulatory, nervous, respiratory and digestive system. Each system receives inputs and releases outputs to other systems which can be monitored by wearable devices throughout the day. Analysis of these inputs/outputs such body temperature and heart rate can to some extent predict the health of an individual, diagnose possible conditions and assist clinicians with therapeutic suggestions.40 The integration of machine learning into these technologies may aid in streamlining this process, by identifying and learning patterns in these signals, ultimately leading to personalised healthcare tailored to the individual.
Researchers are currently investigating how AI can be used to extract medical information from individuals, so that clinicians can make more informed decisions for their patients. This technology combining wearable sensors with machine learning has potential applications in developing countries and low-socioeconomic communities where access to medical personnel and testing equipment for diagnosis is hard to come by. Assessing an individual’s risk for developing a cardiovascular disease or of experiencing a heart attack will aid in reducing mortality rates and enhancing the power of early diagnosis for prevention of these events. If predictions are made well in advance, clinicians can adapt their diagnosis and treatment regimen on a patient by patient basis.41
Whilst digital healthcare tools are continuously evolving, current systems are unable to efficiently cope with the exponential growth in data needing to be stored and extracted, leading to major difficulties in analysing it effectively. Modern data analysis approaches, including applying AI and machine learning algorithms for efficient data mining and subsequent analysis, may prove vital in efficiently filtering through the vast libraries of data collected from wearable sensors. By leveraging these technologies, it may become possible to analyse and interpret complex patterns in collected data, thereby extracting only the relevant biological signals that might otherwise remain hidden within the sheer volume of raw data.42 Through applying machine learning predictive algorithms, AI can help in detecting patterns indicative of cardiovascular health conditions, facilitating tailored healthcare management and personalised insights into the health of an individual.
Future advances and applicability of the technology
Although various challenges exist in regard to the applicability, accuracy and usability of wearable devices, this technology still provides promising opportunities for research into the treatment and monitoring of CVDs. Particularly in regards to asymptomatic indications of cardiovascular diseases such as arrythmias (irregular heartbeats), tachycardia (slow heart beats < 60 bpm) and brachycardia (fast heart beats > 100 bpm), these technologies may become vital.40 In order to introduce these devices into clinical practices, their accuracy and usability need to be verified through large-scale prospective cohort clinical trials with well-established experimental protocols. This would enable the relationship between wearable device data and clinical outcomes such as the early detection of arrythmias, coronary heart disease and strokes to be established.32
Whilst wearable sensors hold much promise for the advancement of healthcare in decades to come, data privacy and security concerns are a pressing issue that must still be addressed. Without adequate safeguards in place, the proliferation of wearable sensors could inadvertently lead to breaches of individuals' privacy and the unauthorised disclosure of sensitive health information. As these devices collect and transmit sensitive health information, including physiological data and activity patterns, they become lucrative targets for cyberattacks and unauthorised access. Vulnerabilities in IoT devices, such as weak encryption protocols or inadequate authentication mechanisms, can expose individuals' personal health data.
Moreover, the interconnected nature of IoT ecosystems increases the risk of data breaches, as compromised devices may serve as entry points to the larger network, where vast amounts of patient data are stored. Furthermore, concerns regarding data ownership and consent arise, as users may not fully understand how their data is being collected, stored and shared by wearable sensors and IoT devices. Addressing these privacy and security challenges requires robust encryption protocols, stringent access controls and transparent data governance practices to safeguard individuals' privacy rights and ensure the confidentiality and integrity of their health information.
Integration into a clinical setting
The integration of wearable sensors into a clinical setting holds immense potential to enhance patient monitoring, diagnosis and treatment. Through providing real-time data on biometric signals and activity levels and other physiological parameters, wearable sensors can enable healthcare providers to gain valuable insights into patients’ health status without the need for a clinical setting. By instigating this remote approach to cardiovascular care, clinicians can monitor the status of patients with chronic or ongoing conditions, track post-surgery recovery and can adjust treatment plans based on the data they receive.
However, several challenges must first be addressed before these devices can be effectively integrated into a clinical setting. Firstly, the accuracy and reliability of the data obtained from patient owned sensors must be validated through gold-standard clinical trials. In addition to this, protocols in regard to patient privacy and data security must be rigorously followed to ensure compliance with legal regulations and ethical standards. Furthermore, clinics and healthcare providers may face scrutiny or encounter resistance from patients, especially those in the elderly population who are not as well versed in technology as younger generations. Over the new few years, we can expect to see some of these challenges overcome as healthcare organisations, regulatory bodies and medical device and technology developers begin to collaborate and leverage artificial intelligence, big data and analytics to enhance the interoperability of these devices.
Conclusions
The landscape of CVD management is poised for significant transformation with the recent emergence of easy to use, highly sensitive and cost-effective wearable sensor technology. The integration of artificial intelligence, machine learning and big data analytics has the potential to revolutionise the prevention, diagnosis and treatment of CVD without the need for a clinical setting.
Wearable sensors, such as the ATsens patch and Oura Ring, are equipped with advanced biometric sensors, enabling real-time detection of physiological parameters, promoting an individualistic and proactive at-home approach to monitoring of CVD. Furthermore, the innovative design of these devices may aid in the early detection of CVD, decrease mortality rates worldwide and lower the cost of healthcare for individuals from low socioeconomic backgrounds suffering from chronic cardiovascular conditions. Continued research, innovation and collaboration between various scientific disciplines will be paramount in unleashing the full potential of wearable sensor technology for the treatment of cardiovascular disease.
References
- Wilson, N.; Cleghorn, C.; Nghiem, N.; Blakely, T. Population Health Metrics 2023, 21 (1), 1. DOI: 10.1186/s12963-023-00301-1.
- Muhammad, Y.; Tahir, M.; Hayat, M.; Chong, K. T. Scientific Reports 2020, 10 (1), 19747. DOI: 10.1038/s41598-020-76635-9.
- Yan-Bo, Z.; Xiong-Fei, P.; Chen, J.; Cao, A.; Lu, X.; Zhang, Y.; Wang, J.; Li, H.; Liu, G.; Pan, A. Journal of Epidemiology and Community Health 2021, 75 (1), 92-99. DOI: https://doi.org/10.1136/jech-2020-214050 Natural Science Collection; ProQuest One Academic.
- Lin, J.; Fu, R.; Zhong, X.; Yu, P.; Tan, G.; Li, W.; Zhang, H.; Li, Y.; Zhou, L.; Ning, C. Cell Reports Physical Science 2021, 2 (8), 100541. DOI: https://doi.org/10.1016/j.xcrp.2021.100541.
- Jensen, M. T. Scandinavian Journal of Clinical and Laboratory Investigation 2019, 79 (1-2), 108-116. DOI: 10.1080/00365513.2019.1566567.
- Lubrano, V.; Balzan, S. World J Exp Med 2015, 5 (1), 21-32. DOI: 10.5493/wjem.v5.i1.21 From NLM.
- Vijayalakshmi, A.; Jose, D. V.; Unnisa, S. Wearable Sensors for Pervasive and Personalized Health Care. In IoT in Healthcare and Ambient Assisted Living, Marques, G., Bhoi, A. K., Albuquerque, V. H. C. d., K.S, H. Eds.; Springer Singapore, 2021; pp 123-143.
- Haq, I. U.; Chhatwal, K.; Sanaka, K.; Xu, B. Vascular Health and Risk Management 2022, 18 (null), 517-528. DOI: 10.2147/VHRM.S279337.
- World Health Organization, Aging and health. 2022. https://www.who.int/news-room/fact-sheets/detail/ageing-and-health (accessed 27/02/2024).
- 10. Baig, M. M.; Afifi, S.; GholamHosseini, H.; Mirza, F. Journal of Medical Systems 2019, 43 (8), 233. DOI: 10.1007/s10916-019-1365-7.
- Park, J.-H.; Jang, D.-G.; Park, J. W.; Youm, S.-K. Sensors 2015, 15 (9), 23402-23417.
- Prieto-Avalos, G.; Cruz-Ramos, N. A.; Alor-Hernández, G.; Sánchez-Cervantes, J. L.; Rodríguez-Mazahua, L.; Guarneros-Nolasco, L. R. Biosensors 2022, 12 (5), 292.
- World Health Organization. Global Strategy on Human Resources for Health: Workforce 2030, 2016.
- Health New Zealand Te Whatu Ora Health Workforce Plan 2023/24; 2023.
- Hitchon, E. G. D.; Eggleston., K.; Mulder, R.; Porter, R.; Douglas, K. New Zealand Medical Journal 2024, 137 (1592). DOI: 10.26635/6965.6553.
- Williams, G. J.; Al-Baraikan, A.; Rademakers, F. E.; Ciravegna, F.; van de Vosse, F. N.; Lawrie, A.; Rothman, A.; Ashley, E. A.; Wilkins, M. R.; Lawford, P. V.; et al. The Lancet Digital Health 2023, 5 (7), e467-e476. DOI: 10.1016/S2589-7500(23)00087-0 (acccessed 13/02/2024).
- Ates, H. C.; Nguyen, P. Q.; Gonzalez-Macia, L.; Morales-Narváez, E.; Güder, F.; Collins, J. J.; Dincer, C. Nature Reviews Materials 2022, 7 (11), 887-907. DOI: 10.1038/s41578-022-00460-x.
- Katrukha, I. A.; Riabkova, N. S.; Kogan, A. E.; Vylegzhanina, A. V.; Mukharyamova, K. S.; Bogomolova, A. P.; Zabolotskii, A. I.; Koshkina, E. V.; Bereznikova, A. V.; Katrukha, A. G. Clinica Chimica Acta 2023, 542, 117281. DOI: https://doi.org/10.1016/j.cca.2023.117281.
- CD, C. Clinical Methods: The History, Physical, and Laboratory Examinations; Butterworths, 1990.
- Ridker, P. M.; Bassuk, S. S.; Toth, P. P. Current Atherosclerosis Reports 2003, 5 (5), 341-349. DOI: 10.1007/s11883-003-0004-3.
- Thygesen, K.; Mair, J.; Katus, H.; Plebani, M.; Venge, P.; Collinson, P.; Lindahl, B.; Giannitsis, E.; Hasin, Y.; Galvani, M.; et al. European Heart Journal 2010, 31 (18), 2197-2204. DOI: 10.1093/eurheartj/ehq251 (acccessed 14/03/2024).
- Yamamoto, Y.; Yamamoto, D.; Takada, M.; Naito, H.; Arie, T.; Akita, S.; Takei, K. Advanced Healthcare Materials 2017, 6 (17), 1700495. DOI: https://doi.org/10.1002/adhm.201700495.
- Oy, Ō. H. 2024. https://ouraring.com/ (accessed 14/03/2024).
- Lee, M.; Yoo, M. Sensors and Actuators A: Physical 2002, 96 (2), 97-104. DOI: https://doi.org/10.1016/S0924-4247(01)00769-5.
- Team, Ō. H. Technology in the Oura Ring. 2024. https://ouraring.com/blog/ring-technology/ (accessed 14/03/2024).
- Surantha, N.; Atmaja, P.; David; Wicaksono, M. Procedia Computer Science 2021, 179, 936-943. DOI: https://doi.org/10.1016/j.procs.2021.01.083.
- Sun, Y.; Song, H.; Jara, A. J.; Bie, R. IEEE Access 2016, 4, 766-773. DOI: 10.1109/ACCESS.2016.2529723.
- Adi, E.; Anwar, A.; Baig, Z.; Zeadally, S. Neural Computing and Applications 2020, 32 (20), 16205-16233. DOI: 10.1007/s00521-020-04874-y.
- Chandrabhatla, A. S.; Pomeraniec, I. J.; Ksendzovsky, A. npj Digital Medicine 2022, 5 (1), 32. DOI: 10.1038/s41746-022-00568-y.
- Singh, P. D.; Dhiman, G.; Sharma, R. Sustainable Computing: Informatics and Systems 2022, 33, 100622. DOI: https://doi.org/10.1016/j.suscom.2021.100622.
- Boyd, D.; Crawford, K. Communication & Society 2012, 15 (5), 662-679. DOI: 10.1080/1369118X.2012.678878.
- Miao, F.; Wu, D.; Liu, Z.; Zhang, R.; Tang, M.; Li, Y. Chin Med J (Engl) 2023, 136 (9), 1015-1025. DOI: 10.1097/cm9.0000000000002117.
- Baek, S.; Ha, Y.; Park, H.-w. PM&R 2021, 13 (4), 379-386. DOI: https://doi.org/10.1002/pmrj.12424.
- de Moraes, J.; Id, M.; Rocha; Vasconcelos, G.; Vasconcelos Filho, J. E.; Hugo, V.; Albuquerque, V.; Alexandria, A. Sensors 2018, 18. DOI: 10.3390/s18061894.
- Gillinov, S.; Etiwy, M.; Wang, R.; Blackburn, G.; Phelan, D.; Gillinov, A. M.; Houghtaling, P.; Javadikasgari, H.; Desai, M. Y. Medicine & Science in Sports & Exercise 2017, 49 (8).
- Li, Q.; Clifford, G. D. Physiological Measurement 2012, 33 (9), 1491. DOI: 10.1088/0967-3334/33/9/1491.
- Satija, U.; Ramkumar, B.; Manikandan, M. S. IEEE Reviews in Biomedical Engineering 2018, 11, 36-52. DOI: 10.1109/RBME.2018.2810957.
- Zhang, C.; Zhao, Y.; Xu, X.; Xu, R.; Li, H.; Teng, X.; Du, Y.; Miao, Y.; Lin, H.-c.; Han, D. Nature Nanotechnology 2020, 15 (8), 709-715. DOI: 10.1038/s41565-020-0699-0.
- Dwivedi, S.; Purohit, P.; Misra, R.; Pareek, P.; Goel, A.; Khattri, S.; Pant, K. K.; Misra, S.; Sharma, P. Indian Journal of Clinical Biochemistry 2017, 32 (4), 374-398. DOI: 10.1007/s12291-017-0688-8.
- Sabry, F.; Eltaras, T.; Labda, W.; Alzoubi, K.; Malluhi, Q. J. Health. Eng. 2022, 2022, 4653923. DOI: 10.1155/2022/4653923.
- Arunachalam, S. K.; Rekha, R. Concurrency and Computation: Practice and Experience 2022, 34 (19), e7027. DOI: https://doi.org/10.1002/cpe.7027.
- Chen, C.; Ding, S.; Wang, J. Nature Medicine 2023, 29 (7), 1623-1630. DOI: 10.1038/s41591-023-02391-8.